With advancing computational technology and modern training algorithms, there is a surge of interest in Deep Learning. Deep Learning has brought about remarkable breakthroughs in speech and image recognition and is producing advances in many domains of science.
We are seeking to apply the Artificial Neural Network (ANN), the very core of Deep Learning, and other machine learning techniques to combustion problems. It can easily take advantages of the increasing amount of data from simulations (LES and DNS) and experiments, and thereby help us solve complex problems for which there are no good solutions by means of traditional approaches or help us discover new patterns and structure in large data sets. For example, multi-layer ANNs (represented in Fig. 1) were trained with Google’s TensorFLow and used to predict CO-LIF signals of Dimethyl ether (DME) flames (Fig. 2). Another application is to predict collision probabilities of clusters in modeling the agglomeration of nanoparticles (Fig. 3).
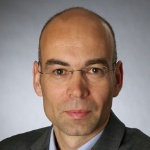
Andreas Kronenburg
Univ.-Prof. Dr.Director of the Institute
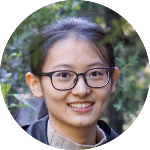
Ruyue Cheng
Scientific staff